Part II – New Model Army
In Part I of the inquiry, I set out some of the structural problems that the Finance Industry is facing in the wake of the macroeconomic adjustments we experienced over the last 18 months or so and the impact that they have had on portfolio returns both in 2022 and in the future. In part II I take a closer look at the range of investment approaches – from passive to active currently being operated in the market and at the types of investment models underpinning them.
From Passive to Active
The broad underpinnings of Passive investing are hardly controversial: a belief in markets being essentially efficiently priced, a focus upon systematic risk, diversification of stock level risk across a relatively large number of individual holdings and an approach towards reducing overall risk by focusing on lowering portfolio level volatility. This essentially “precautionary”, risk-managed approach to investment is clearly at odds with the Active “return maximising” approach, with its focus upon finding potential mispricing in markets and the active embracement of stock level risk via relatively concentrated portfolios (and hence at attempt to maximise returns) in order to beat the market benchmark. These are clearly two quite different approaches to portfolio management and, I discussed in part 1, it is clear that more Passive funds are facing some clear structural problems going forward. However, that does not mean that Active funds are immune from pressure – both approaches will need to be made “fit for purpose” going forward.
On the spectrum…
Much has been made of the supposedly binary split between active and passive investment styles – often summarised as “betting against market prices versus embracing them” – with each camp’s champions seeking to decry the validity of the other, but the reality is that the debate has moved on and end investors are increasingly taking a more nuanced approach to investment. This reflects the reality on the ground; that there is a spectrum of investment approaches – from Passive to Active – where the focus upon risk management (driven by expected returns) is balanced by the focus upon future price action (driven by excess returns). How one positions a particular investment approach along this spectrum reflects the trade-off required, not only from an investor return point of view but also from an operational perspective. The table below summarises how these may vary from one end of the “spectrum” to the other. If the current structural challenges facing existing products are to be overcome, then any resulting new products will not only need to be operate more effectively in a changing economic and investment climate, they will also need to take these operational requirements into account and optimise them for both institutional and investor benefit in the future. It is to this combined challenge that we now turn.
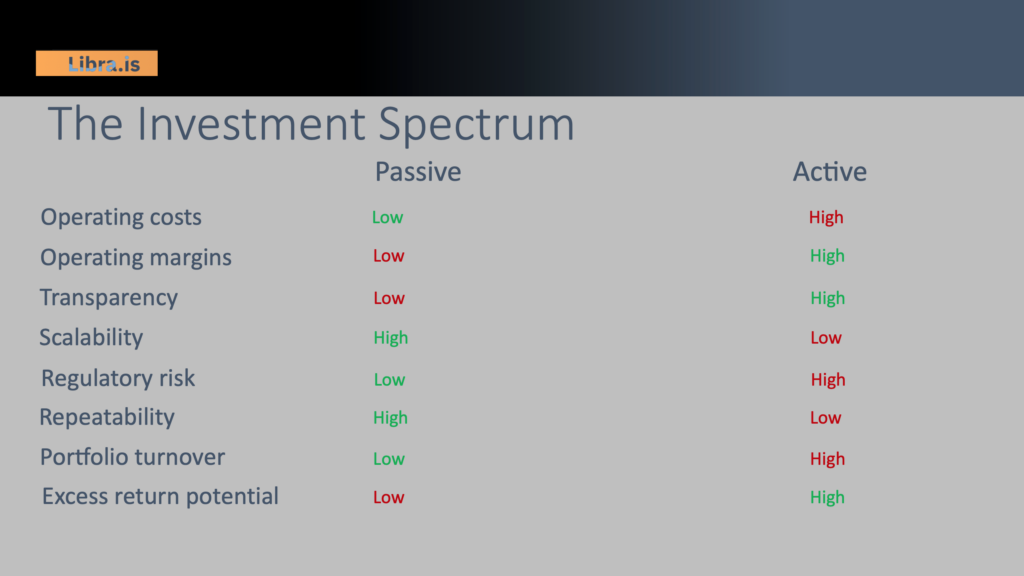
Building the brave new world
Statistical models have had a bad rap in the last few years with their performance in regard to the COVID pandemic a particular focus of criticism. The aphorism “All models are wrong …” – attributed to the statistician George Box – is often presented as a laconic dismissal of the value of economic or mathematical models in general and, even in its fuller form – “All models are wrong, but some are useful” is used to emphasise the essentially limited benefit perceived to be added by such processes. However, it is this “usefulness” that we actively need to engage with. This not only requires an understanding of the differences between various types of model – descriptive models, analytical models, forecast and predictive models and, ultimately, prescriptive decision models – it also requires the application of the right model in the right circumstances.
Putting a portfolio together
When putting an equity portfolio together there are two key elements to the process: asset selection where the focus is upon achieving the forecast outcome / gross return of the combined assets in the portfolio and risk management where the focus is upon managing the risk of not achieving that expected return. Some portfolios are heavily influenced by the former whilst others are biased towards the latter, and the way in which these two elements are combined helps to place a portfolio on the “Investment Spectrum” – from Active (all about forecast outcomes) to Passive (all about risk management). This also means that the types of models used for each of these elements are different in nature, with forecast and predictive models heavily utilised at one end of the spectrum and descriptive and statistical analytical models on the other. However, for the end-result – the portfolio model – to be effective, it needs to be a prescriptive, decision-making model that takes both elements into account and delivers the required outcome. In other words, all forms of model need to feature in a successful portfolio construction process. If a prescriptive model (the portfolio model itself) is only derived from one group of models then we risk retuning to the structural problems of the past.
Analyse…
Much of the traditional analytical work done in the finance industry has been directed towards understanding how price mechanisms work, how information dynamics impact on expectations and how common factors such as value or momentum account for returns across a range of assets. Descriptive models work well in terms of establishing prior relationships – sector-based, thematic or factor-based models for example – and integrate into structural analysis based around price dynamics with respect to these descriptive model characteristics. However, these are statistical analytical models and are generally not forecast models: they may involve time series data and various statistical techniques typically involved in forecasting but are mostly designed to validate and confirm informational relationships in order to construct operational systems.
Forecast…
Forecast based and Predictive models are very different. Rather than basing decisions around point data derived from long run statistical datasets (period average returns for example) what we might call “risk reducing decision models” use baseline forecasts and probability-based analytical techniques to create a more optimal forward guidance type dataset. By using current and historic data to build up relational models, future-focused, probability-based models can be developed that are sufficiently reliable and robust to help model and forecast what might happen in the future based upon a stochastic past. These outputs can be then incorporated into more prescriptive decision-making models to produce the range of decision options and their associated risks upon which a portfolio can be based.
Decide…
This might sound obvious, but if stock selection is done via statistical models based on average historic returns or probability forecasts are based upon simple long run measures of price volatility, then the inputs are going to lead to poor prescriptive outcomes. Similarly, if past descriptive and relational datasets are not sufficiently incorporated when constructing Factor based risk models or managing correlation risk at the portfolio level, then even relatively sophisticated model processes can lead to poor real-world outcomes. This is especially the case where these processes become more automated in nature, often less transparent in approach and where both data quality and model selection are critical.
This might sound obvious, but if stock selection is done via statistical models based on average historic returns or probability forecasts are based upon simple long run measures of price volatility, then the inputs are going to lead to poor prescriptive outcomes.
Remind me – why did you have Amazon in the portfolio?
The widespread adoption of complex AI based modelling and Machine Learning algorithms in financial market systems has led to an increasing need for “explainability and interpretability” of these kinds of model-based approaches. Whilst not the same as “trustworthiness” in the process (one is often misrepresented as the other) there is no doubt that investor buy-in is helped by increased transparency in the underlying methodology and analysis. However, even when able to successfully explain a complex process, sometimes a simple sanity check is still required.
Can you – as an Investment professional – confidently answer the question “…why is (isn’t) Amazon in your portfolio? What part of your portfolio decision making led to that decision and how does that square with the reality that the end investor is able to observe in the real world?
It’s certainly been a relevant question over the last 18 months, as the Chart below shows:
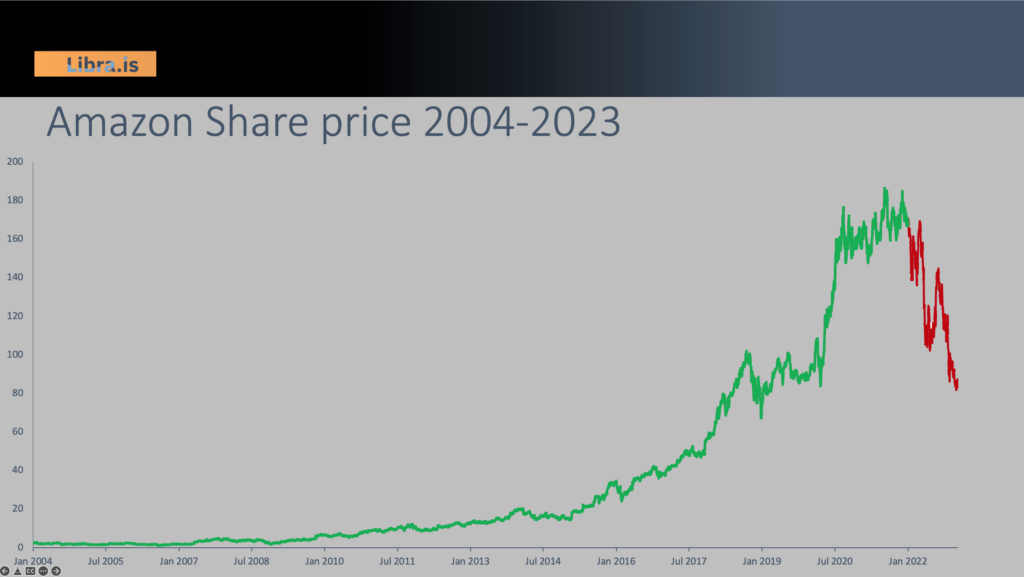
Everyone can see (with hindsight) the attraction of the stock throughout the last decade or more – until the reality of 2022’s tightening interest cycle hit home and the share halved in price. However, if you are not able to explain how or why your portfolio process decided to allocate capital (or not) to Amazon, then it may simply be that there was no identifiable or defensible expected return decision or risk decision in your process.
For example, as the largest stock in the sector, Amazon certainly helped to drive the S&P500 Consumer Discretionary sector down 36% last year, but a decision to maintain exposure to that sector would necessarily have required exposure to Amazon by default. It is also worth noting that it is not only in the Consumer Discretionary Sector and the overall S&P500 Index tracker but – as of Jan 2023 – is simultaneously in the iShares S&P100, iShares S&P500 Growth ETF AND iShares S&P500 Value ETFs. So, as an index or factor-based investor, you could confidently answer that it was a) a big cap stock so I had no choice, b) it was a growth stock so I had no choice and now it will be because it is a value stock and I have no choice. Never mind the company outlook, it’s earnings or its fundamentals, the only way in which you could chose to NOT own Amazon going forward, would be to ACTIVELY choose not to do so at the Market Cap or stock level.
So, what is the answer?
The answer we are looking for when asking the “Amazon question” is actually the answer to the question “how internally prescriptive is the portfolio construction process?” Are you relying on an external preselection of the risk management process or the stock selection process? Are you developing internally consistent definitional models (of value and growth for example) or adopting those provided by third parties? If so, how do the elements of your stock selection process and predictive forecast model integrate with the risk management framework? Is the information set you are using for modelling risk management and stock selection internally consistent?
This returns us to the question of why performance might have been so damaging in the past year and is potentially so in the future. Although we now have a whole arsenal of different investment structures available to us, even when stock memberships of these structured products are not completely fixed, the essential nature of most passive or semi passive portfolio construction processes remains analytical and descriptive in nature and hence retains the bias towards the expected repeatability of past performance. This, in turn, means that there has tended to be a lack of predictive modelling involved and, even where it does exist, it may conflict with externally defined frameworks. By contrast, an overly predictive based approach to Active investment management (narrowly focused stock picking in other words) may have led to a poorly defined understanding of the portfolio level risk factors being taken on by the portfolio process, leading to significant portfolio level losses. In either case we can see that the argument can be made for a more adaptive and dynamic approach to portfolio construction that takes “the best of both” approaches and integrates them into a smarter, more effective process for both institutional and investor benefit.
In part III of the inquiry, I will expand on how an integrated approach to investment risk modelling, stock selection and portfolio construction can be designed. This will place a new type of investment strategy at the optimal point on the Investment Spectrum and enable the joint goals of operational and investment return benefits to be achieved. It’s called Smart Alpha.