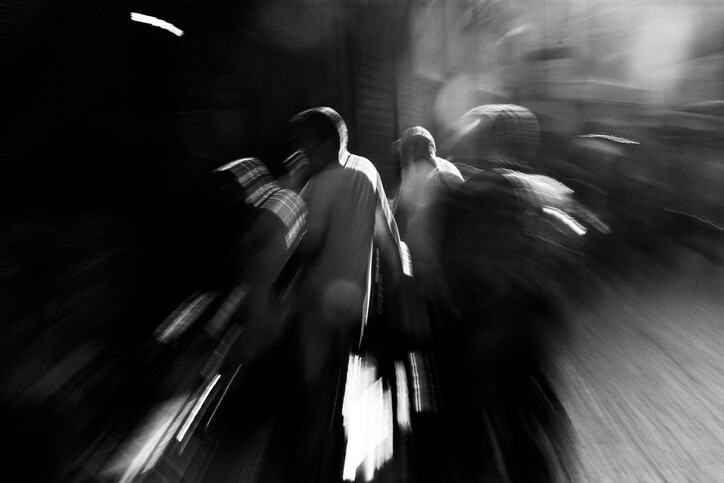
As the (still ongoing) fallout from the market turmoil starts to be evaluated, there has been a natural temptation to rationalise and understand recent activity through the traditional lens of cause and effect:
“… (A) market response to an (un)anticipated event occurred because Event X threatened to provoke subsequent (negative) Outcome Y and markets reacted to – and priced in some/all of the possible downsides of Outcome Y occurring. However, as a subsequent event, let’s call it Event A, occurred that reduced or removed or reduced the likelihood of Outcome Y occurring to an acceptable level such that the status quo can be restored (Outcome B).”
The trouble of course is that there is never one step to the process. One can only say with confidence what happens next if there is a sense to which the events and outcomes being presented can be rationalised and explained sufficiently to be sure of the ongoing outcomes and be confident in the idea of a restoration to a prior status quo.
What the last week has demonstrated is that when the world wakes up and realises that they (properly) understand neither cause nor effect, then confidence (and certainty) collapse. Add in the singular certainty that there is no longer an observable status quo to return to and the cause-and-effect model can no longer function.
Have you spotted the pattern yet?
To understand why this approach is no longer valid requires some self-critical analysis. Although we continuously frame financial markets as rational and efficient processes, we quickly run into problems when, to return to the example above, Event X does not have a singular outcome in response to its occurrence and Outcome Y does not lead to a singular result. Moreover, the assumption that Event A is independent of Event X is not always clear, so it is also hard to assume that the Outcome Y is not likely to reoccur or that another Outcome B is going to occur that is different again. When neither Event X or Event A are expected but do occur but neither Outcome Y or Outcome B follow suit, then we find ourselves waiting for yet another Event to occur (as opposed to an outcome) that might help to rationalise things – and remain paralysed with fear until it does.
This may all sound a bit complicated, but in simple terms it is all about one thing: pattern recognition. Pattern recognition is at the heart of the cause-and-effect review process and is the essence of how most – if not all market trading and activity operates. AI (in its current form at least) is, of course, simply a highly advanced form of pattern recognition, and the more it gets integrated into our day-to-day lives, the more dependent we are becoming on pattern recognition for our decision making.
What this means is that it is not only the complexity of a situation that’s at issue here – it’s the approach one takes towards it. One-size does not fit all, and so it is understanding when it’s not a complex (complicated) problem to be solved –but that it’s an event within a complex, adaptive system to be understood and managed that is critical. Last week’s events in the financial markets are a classic example of such a reality check: we are in the latter scenario. We are literally in ‘Mike Tyson’ territory – where everyone’s plan must be reorganised now that they have been “punched in the face”.
What if there is no pattern to recognise?
For be in no doubt – this is not a problem to be solved – it is a complex systemic shock to be understood and then managed. In that sense there are no simple answers, no simple explanations for behaviours by politicians, corporates, or financial markets, either now or in the past. Suddenly, there are no patterns to easily recognise, revert to or have confidence in. Uncertainty has exploded upwards precisely because not only have individual patterns of behaviour broken down, the assumption of implied, stable, cross-sectional correlations and patterns of behaviour has also been broken.
We are in the hallucination zone
At this point we are most prone to the problem that faces AI: hallucination. AI’s critics rightly point to the problem of hallucination (AKA “…making stuff up”) in the results often provided by open-source AI models and advise against “trusting without verifying” results provided seemingly so effortlessly by our AI enabled information systems. AI models hallucinate for the simple reason that, as pattern recognition systems, they observe actual data (from training data sets for example) but “guess” at what information is missing in a real-world context. Ask an AI bot for an answer to something and it will give it to you – even if it has just made that answer up. Ask human observers to explain what has just happened and they will tend to “fill in the blanks” in order to both narrate and rationalise both past and future. As a result, much of the current commentary around the past week’s events is taking the form of hallucination. Although not fully understanding (or predicting) either cause or effect, there remains an instinct to try to ex-post rationalise and continue to predict future events using an existing cause and effect paradigm.
What’s new?
Complex adaptive systems in general develop what are known as “emergent properties” that are mostly stable but do break down and allow new properties emerge. Whether you think (and whatever you think of) the decisions made by the White House were directed at trade, the bond, equity or currency markets, China, Multinationals, US domestic politicians, central banks or anyone else are not the key issues to be rationalised and understood. What is important is that, just as we saw with International Economic events such as the COVID shutdown, Global geopolitical events such as the war in Ukraine or market specific events such as the GFC, recent events will impact across the whole economic system – including the financial markets in a way that was not previously anticipated and in a manner by which new, complex relationships will emerge.
Ad Homonym attacks on the individuals involved may make headline writers feel good about themselves, but what is important now is to understand what has changed and how things may begin to evolve and what “new” system properties might emerge. What we can say is that behavioural patterns in the complex system(s) of 2024 will be only an historic guide to likely behavioural patterns in 2025 and further out into the future. This is not just the case for those parts of the real and financial sectors that have been most directly affected by recent events. Areas that appear not to have been directly impacted are also likely to see previously unanticipated change. For example the current reality of “service” pricing of IP and transfer pricing by Amazon, Google and other multinationals is not going to survive this – even if there are no formal Tariffs being applied to Services. Chinese based supply chains will face a permanent shift when compared to the past, but no one can evaluate the degree of this or the impact of second-round effects on issues such as the retaining or licensing of IP or the impact on transportation, shipping or delivery channels as yet.
Similarly, as the focus shifts on from the short-term investment return impacts, structural issues relating to market liquidity, risk management and regulation will emerge. Financial markets will experience longer term shifts in risk appetite towards risk assets, fixed income, investment themes, geographical and economic exposure whilst capital market structure and market liquidity concerns may lead to regulatory and policy responses that are currently unknown. All of this will have ongoing system impacts and implications for wealth products, Active/Passive investments, fractional tokenisation of Real-World-Assets (RWAs) and a myriad of other possibilities that we can only guess at for now.
The information solution
Having made the point that pattern recognition models have broken down it is worth noting that not ALL pattern recognition approaches will have done so. Financial market models that depend on realised patterns of past behaviour being effective forecasts of the future are the models most in crisis. Assumptions of mean reversion to historical norms or averages implode in the face of such pattern breaks and take time to rebuild. This is prime territory for hallucination ,as confidence in the performance of the pattern can be cited “…so long as” the assumption of a return to normal can be asserted as likely. What marks such models out is the implicit assumption that uncertainty (volatility, risk or whatever you define it by) tends to be stochastic and mean reverting to zero over time. Uncertainty in other words, is not part of the model parameters and it is only when uncertainty subsides and markets begin to re-establish some form of price equilibrium with respect to fundamentals that such models have any value.
The pattern recognition models that are far less likely to breakdown are those where uncertainty – in the form of uncertainty relating to both forecast returns and the risk-pricing of those returns, are intrinsic to the pattern recognition process. It should not come as a surprise to those who are familiar with our work or our data to know that implied volatility with respect to both facets of the classical asset pricing model are key components that we employ in the smart alpha strategic models that we run and generate strategic advice from. These implied volatility forecasts are themselves the outputs of our predictive time-series modelling of uncertainty at the stock level and are available as downloadable time series data from Libra.
If you are interested in building our “non-hallucinating” models for global equity markets, then you might just find it a data set worth employing.